Webinar video https://www.youtube.com/watch?v=zFxwgsoS58g
Presentation slides (direct link)
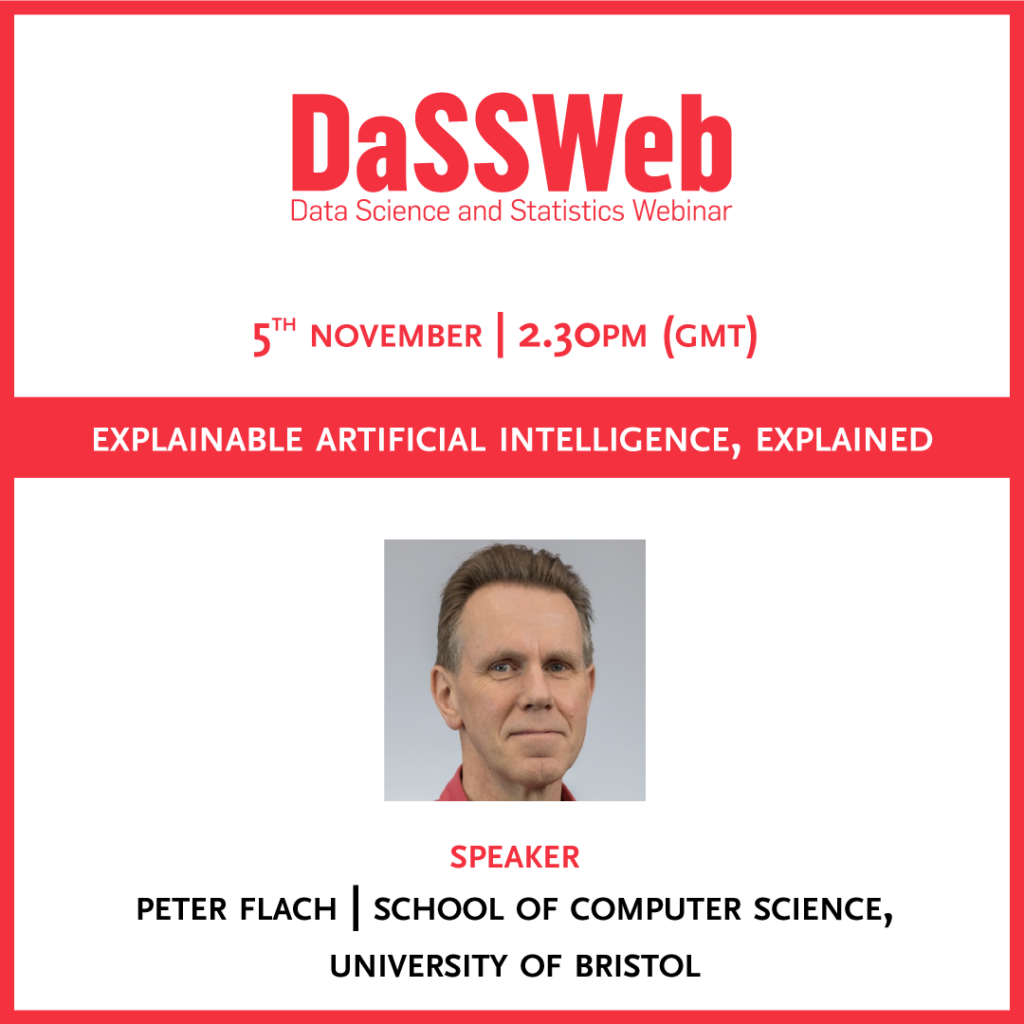
Abstract
Explainable Artificial Intelligence (XAI for short) aims at giving insight in the behaviour of AI models in general and machine learning models in particular. In this talk I will give an overview of this growing field, using some recent results from my group as examples. These include explainability for time series (LIMEsegment); actionable counterfactuals (FACE); explainability fact sheets; as well as the fat-forensics.org toolkit for evaluating Fairness, Accountability and Transparency of AI systems. Finally, I will discuss the importance of properly treating probabilities in feature attribution methods such as LIME and SHAP through log-linear models, and extensions to multi-class settings.
Short Bio
Peter Flach is Professor of Artificial Intelligence at the University of Bristol since 2003. An internationally leading scholar in the evaluation and improvement of Machine Learning models, he has also published on mining highly structured data, on knowledge-driven and explainable AI, and on the methodology of Data Science. He is author of many books, one which has, to date, sold over 20,000 copies and has established itself as a key reference in Machine Learning, with translations into Russian, Mandarin, and Japanese. Prof. Flach was, until 2020, Editor-in-Chief of the Machine Learning Journal, and has been chair of the main international conferences in the area. He is a founding board member, previous President and current Vice-President, of the European Association for Data Science. He is a Fellow of the European Lab for Learning and Intelligent Systems, and of the European Association for Artificial Intelligence. Prof. Flach has a strong track record of collaborations both in academia and in industry.
Information at FEP News
More information at News U. Porto